In prior weeks, we’ve reviewed DHT companies by therapeutic area and introduced the idea of composability when combining hardware and software measures. This week, we walk you through the process of selecting measures that matter using HumanFirst's unique Measure Ontologies.
Precision Measures that Matter
As an academic researcher in rehabilitation, I was constantly searching for digital health technologies that could measure meaningful aspects of health across various domains.
A question like “does a sensor that can measure sleep, arm movements, activity, and social interactions, plus provide raw data, actually exist?” could mean weeks or months of research — and even then I’d never know for sure if other, better options were available.
One of my goals when I joined HumanFirst was to help other researchers better understand the tradeoffs among digital measures, making it easier to develop study endpoints that are safe, effective, and meaningful. Though the digital health technology space is large and ever-evolving, I’ve found Atlas to be the single best tool available to identify measures that matter.
Developing Trade-Off Scenarios
Finding the ‘perfect’ combination of digital health technologies and data platforms to simultaneously gather all desired measures of interest for a clinical trial can be challenging. HumanFirst’s Atlas platform makes it simpler to weigh trade-offs based on the latest data, curated from thousands of published research papers, clinical trials, and more!
For example, imagine you are interested in “axillary body temperature,” and the digital health technology needs to be cleared for clinical use in the Unites States (FDA registered). How would you start generating trade-off scenarios?
Atlas’s Measure Ontologies do this work for you:

In Atlas, you can evaluate by a variety of factors at a glance, including:
1. Ability to capture measures in a specific population
2. Related regulatory decisions (e.g., clearances and/or use in other trials)
3. Dozens of technical specifications
Let’s walk through how this process works with a specific example.
Sleep and Activity in Stroke Patients
Say you are designing a trial that needs to measure sleep and activity in stroke patients. You can see from Atlas that Fitbit is among the top vendors in related measure categories (as ranked by quantity of evidence — learn more here) and you have some experience with their Inspire 2, so you decide to start there.
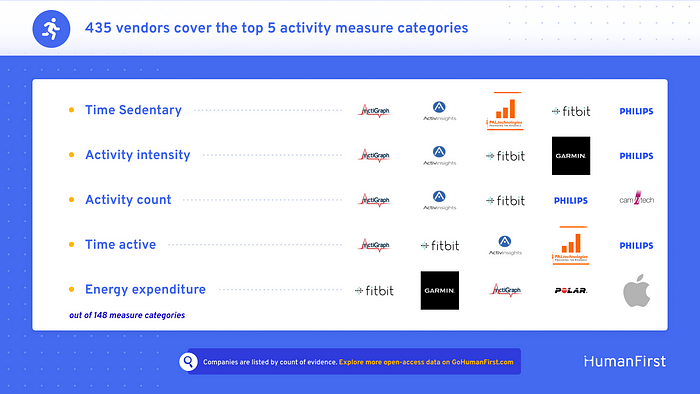
Now assume that your study requires technology that is cleared for use in the European Union (CE-marked), has been used successfully in patients with stroke, and it would certainly make your life easier if it had an API. Does your chosen technology fit all of these criteria?
Based on the Atlas platform's filtering and comparison tools, it looks like the Fitbit Inspire 2 is a great option, with support for all of your measures of interest, a history of use in the target population, and an available API.
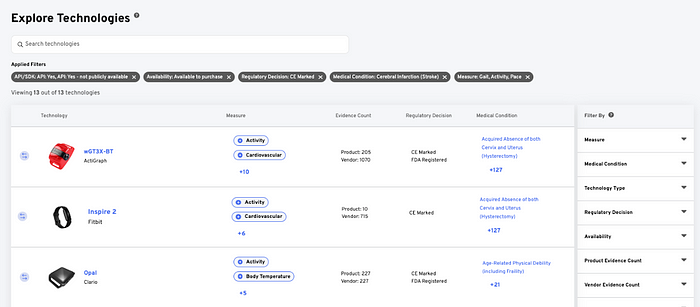
Another way to approach this question would be starting first from one of the digital measures of interest — in this case, the concept of “Sleep”.
Atlas’s Measure Ontologies would quickly highlight that “Sleep” is actually a broad category, encompassing hundreds of underlying measures classified in 10+ subcategories such as:
- Sleep stages: 100+ digital measures
- Sleep quality: 40+ digital measures
- Activity during sleep: 125+ digital measures
In total, this single concept is tied to more than 1,100 reported measures.
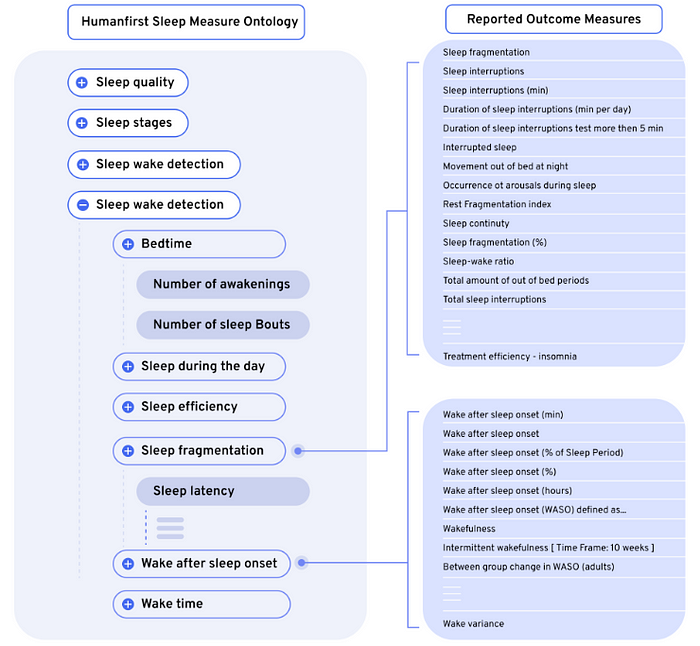
You previously identified the Fitbit Inspire 2 as a digital health technology with good coverage of sleep and activity measures. With Measure Ontologies, we identify two related measures — “Sleep onset” and “Wake after sleep onset” — that would be a valuable addition to our study. Unfortunately, the Inspire 2 doesn’t appear to have any evidence in these categories.
Within moments, however, you use Atlas to see that another DHT, ActiGraph’s wGT3X-BT, meets all of your criteria including the sleep onset measures that you’ve just discovered.
Diving in to Measure Ontologies
Curious about other ways that our Measure Ontologies can help you select measures that matter for your study population? Download the above use case along with two more, covering:
- Capturing intra-ocular pressure at home
- Detecting pre-symptomatic COVID-19 with high fidelity
Download now!
Interested in how our Measure Ontologies were constructed? My colleague Christine Campbell has explained the history of these ontologies and how to use them.
Find Measures that Matter to You
Use the slides below to kick off your own digital measure investigations, and sign up for Atlas to access the full list of 15,000+ digital measures.
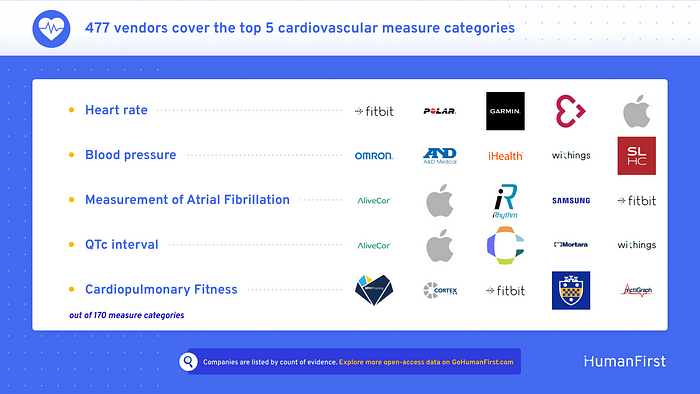
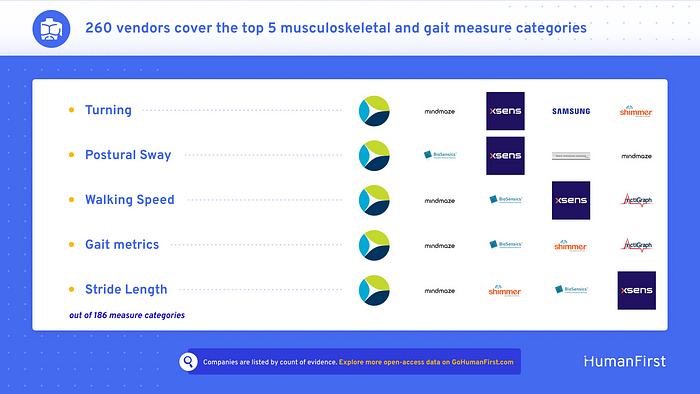
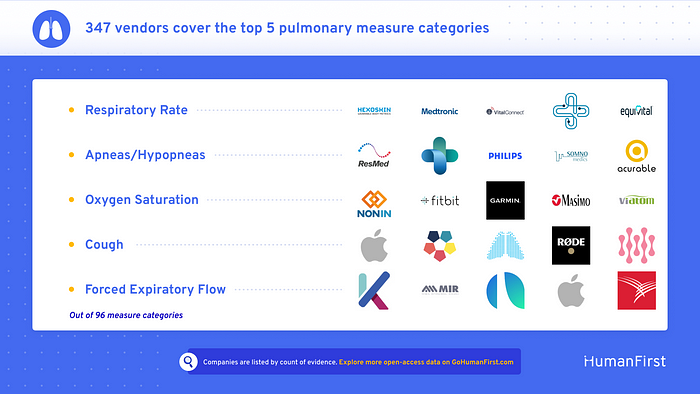
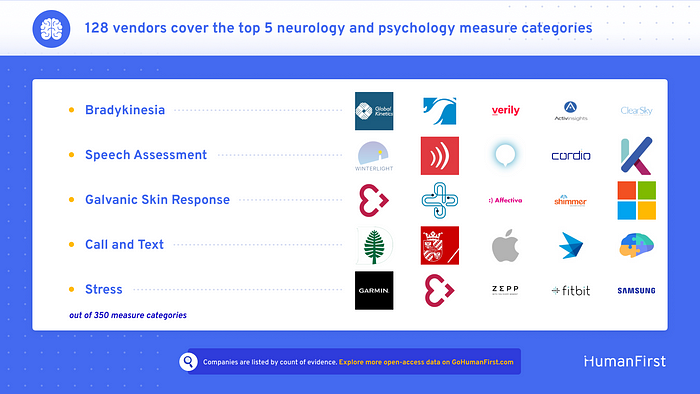
